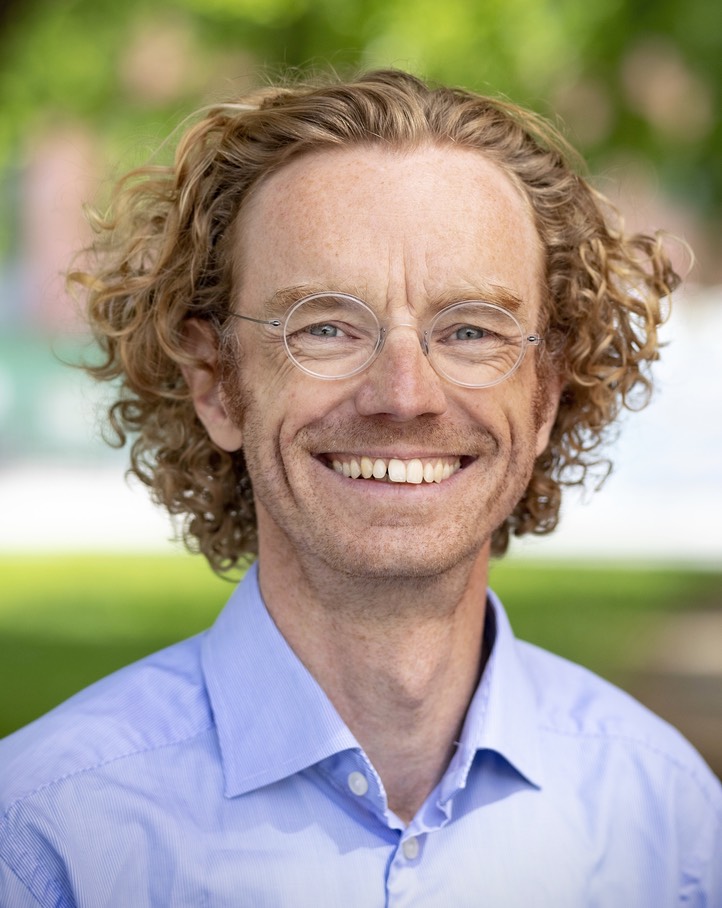
Our aim is to automate the extraction of knowledge and understanding from data. Allowing machines (and humans) to understand what is happening and to acquire new skills and learn new things. We achieve this by developing new probabilistic models and deriving algorithms capable of learnings these models from data. The systematic use of probability in representing and manipulating these models is key. It allows us to represent not only what we know, but to some extent also what we do not know. We take a particular interest in dynamical phenomena evolving over time.
Our research is multi-disciplinary and it sits somewhere on the intersection of the areas of Machine learning and statistics, signal processing, automatic control and computer vision. We pursue both basic and applied research, which explains our tight collaboration with various companies. A slightly more detailed overview of our research is available here.
Recent research results/news
December 20, 2022 [Two tenure track Assisstant Professorships available] In 2023 we are launching the Beijer laboratory for Artificial Intelligence. For this reason we have opened two tenure track Assistant Professorships, one directed towards applications in life sciences [here] and one towards the societal impacts [here]. These positions will extent and complement our existing research and education within artificial intelligence and machine learning.
December 15, 2022 [Short Team update] Dominik Baumann who has been a post-doc in the team for a year will after the Holidays start a tenure track position as Assistant Professor at Aalto University (Espoo, Finland). He will remain affiliated with us and the collaborations we have started will of course continue. During the first half of next year Antonio Ribeiro will spend roughly 3 months in the team of Francis Bach at The Ecole Normale Supérieure (Paris, France) to explore some mutual interests. Daniel Gedon will do his pre-doc with Mikhail Belkin and his team at the University of California San Diego (USA), where he will spend roughly 3 months.
November 28, 2022 [Paper accepted for TMLR] We have been working on incorporating existing background knowledge into Machine Learning models for quite some time and in this paper we provide new results for the multitask Gaussian processes. We show how to include background knowledge in the form of constraints that require a specific sum of the outputs to be constant. This is achieved by conditioning the prior distribution on the constraint fulfillment. The approach allows for both linear and nonlinear constraints.
Philipp Pilar, Carl Jidling, Thomas B. Schön and Niklas Wahlström. Incorporating sum constraints into multitask Gaussian processes. Transactions on Machine Learning Research (TMLR), 2022. [TMLR]
Click here for older news.